ギャップを埋める:深層学習におけるソースフリードメイン適応の力 / Bridging the Gap - The Power of Source-Free Domain Adaptation in Deep Learning
AIの新境地:ソースフリードメイン適応が未ラベルデータの活用を可能に / Revolutionizing AI - How Source-Free Domain Adaptation Streamlines Processes and Reduces Costs
7/27/2023
(English Version Below)
今日の急速に進化する世界では、人工知能(AI)の力がますます複雑な問題の解決、プロセスの効率化、日常生活の向上に活用されています。AIの一分野であるソースフリードメイン適応(SFDA)は、未ラベルのデータのみを使用して事前学習済みモデルを新たなドメインに適応させることで、大きな進歩を遂げています。これにより、計算コストの削減、環境への影響の軽減、最先端のAI技術へのアクセスの民主化が可能となる可能性があります。
Googleの研究科学者であるEleni Triantafillouと学生研究者のMalik Boudiafは、最近のブログ投稿でSFDAの重要性を強調しています。彼らは、深層学習が大きな進歩を遂げている一方で、モデルは見たことのないドメインや分布に適用すると予測不能な失敗をしばしば引き起こすと指摘しています。SFDAは、事前学習済みモデル(ソースドメインで学習したもの)を新たなターゲットドメインに適応させる方法を設計することで、この問題に対する解決策を提供します。
この研究分野は、ターゲットドメインからのラベル付き例の利用可能性が低いという問題を抱えている実際のアプリケーションに対して、大きな実用的な意義を持っています。さらに、大規模モデルの訓練はますます計算コストがかかるようになり、一部の実践者にとっては手の届かないものとなり、環境に対する負荷も増大しています。SFDAは、既に訓練されたモデルを再利用して新たなタスクに取り組むか、新たなドメインに一般化することで、大規模な計算とエネルギー使用の必要性を減らす解決策を提供します。
従来のアプローチから一歩踏み出して、TriantafillouとBoudiafは、自然に発生する分布のシフトが多く、ターゲットのラベル付きデータが不足していることが特徴的なバイオアコースティックスの分野に注目しています。彼らは、この応用でSFDAを研究することが、既存の方法の汎用性について学術界に情報を提供し、新たな研究の方向性を特定し、現場の実践者に直接的な利益をもたらすことができると主張しています。彼らの研究は、これらのシフトに対して既存の方法を上回る性能を発揮し、さまざまな視覚データセットで強力なパフォーマンスを示す新たな方法、NOTELAの開発につながりました。
結論として、SFDAの力は、私たちが日々の生活でAIを使用し、その恩恵を受ける方法を変革する大きな可能性を秘めています。計算コストと環境への影響を減らし、事前学習済みモデルを新たなドメインに適応させることで、SFDAはAI技術へのアクセスを民主化する助けとなっています。この革新的なアプローチの全体像を探求し続ける中で、AIがよりアクセスしやすく、持続可能で、私たちの変わりゆく世界に適応する未来を楽しみにしています。
Bridging the Gap: The Power of Source-Free Domain Adaptation in Deep Learning
In today's fast-paced world, the power of artificial intelligence (AI) is increasingly being harnessed to solve complex problems, streamline processes, and enhance our everyday lives. One area of AI, known as source-free domain adaptation (SFDA), is making significant strides in enabling pre-trained models to adapt to new domains, using only unlabeled data. This has the potential to reduce computational costs, mitigate environmental impacts, and democratize access to cutting-edge AI technologies.
Eleni Triantafillou, a Research Scientist, and Malik Boudiaf, a Student Researcher at Google, have highlighted the importance of SFDA in a recent blog post. They note that while deep learning has made significant advances, models often fail unpredictably when applied to unseen domains or distributions. SFDA offers a solution to this problem by designing methods to adapt a pre-trained model (trained on a source domain) to a new target domain, using only unlabeled data from the latter.
This area of research has significant practical implications as many real-world applications where adaptation is desired suffer from the unavailability of labeled examples from the target domain. Moreover, the training of large-scale models is increasingly computationally expensive, potentially out of reach for certain practitioners and harmful for the environment. SFDA provides a solution by reusing already trained models for tackling new tasks or generalizing to new domains, thereby reducing the need for extensive computation and energy use.
In a departure from traditional approaches, Triantafillou and Boudiaf have turned their attention to the field of bioacoustics, where naturally-occurring distribution shifts are ubiquitous and often characterized by insufficient target labeled data. They argue that studying SFDA in this application can inform the academic community about the generalizability of existing methods, identify open research directions, and directly benefit practitioners in the field. Their research has led to the development of NOTELA, a new method that outperforms existing methods on these shifts while exhibiting strong performance on a range of vision datasets.
In conclusion, the power of SFDA holds significant potential to transform the way we use and benefit from AI in our daily lives. By reducing computational costs and environmental impacts, and allowing for the adaptation of pre-trained models to new domains, SFDA is helping to democratize access to AI technologies. As we continue to explore the full potential of this innovative approach, we can look forward to a future where AI is more accessible, sustainable, and adaptable to our ever-changing world.
参考
[1] https://ai.googleblog.com/feeds/posts/default?alt=rss. "In search of a generalizable method for source-free domain adaptation". 2023-07-26.
[2] Pararawendy Indarjo. "Dealing with Conversion Metrics? Consider Beta-Binomial Model". 2023-07-26.
[3] Adam Ross Nelson. "The Good, The Bad, and the Ugly of Pd.Get_Dummies". 2023-07-26.
[4] Yennie Jun. "Where Are All the Women?". 2023-07-26.
[5] Emily Webber. "New technical deep dive course: Generative AI Foundations on AWS". 2023-07-26.
Other References and Readings
[1] https://ai.googleblog.com/feeds/posts/default?alt=rss. "In search of a generalizable method for source-free domain adaptation". 2023-07-26
[2] Pararawendy Indarjo. "Dealing with Conversion Metrics? Consider Beta-Binomial Model". 2023-07-26
[3] Adam Ross Nelson. "The Good, The Bad, and the Ugly of Pd.Get_Dummies". 2023-07-26
[4] Yennie Jun. "Where Are All the Women?". 2023-07-26
[5] Emily Webber. "New technical deep dive course: Generative AI Foundations on AWS". 2023-07-26
免責事項:このサイトのコンテンツは、精巧に作られたプロンプトに基づいて人工知能によって生成されています。私たちが使用しているテクノロジーは、正確でタイムリーな情報を提供することを目指して設計されています。しかし、高品質のコンテンツを提供することを目指している一方で、人工知能システムが人間のように内容と文脈を完全に理解することはできないという点を明記しておきます。提供される情報は、あくまでご自身の調査や専門家との相談の出発点として使用するべきであり、意思決定の唯一の根拠として依存すべきではありません。
Disclaimer: The content on this site is generated by artificial intelligence based on carefully crafted prompts. The technology we use is designed to provide accurate and timely information. However, while we aim to provide high-quality content, it is important to note that the artificial intelligence system does not fully understand the content and context in the way that a human does. The information provided should be used as a starting point for your own research or consultation with a professional, and should not be relied upon as the sole basis for making decisions.
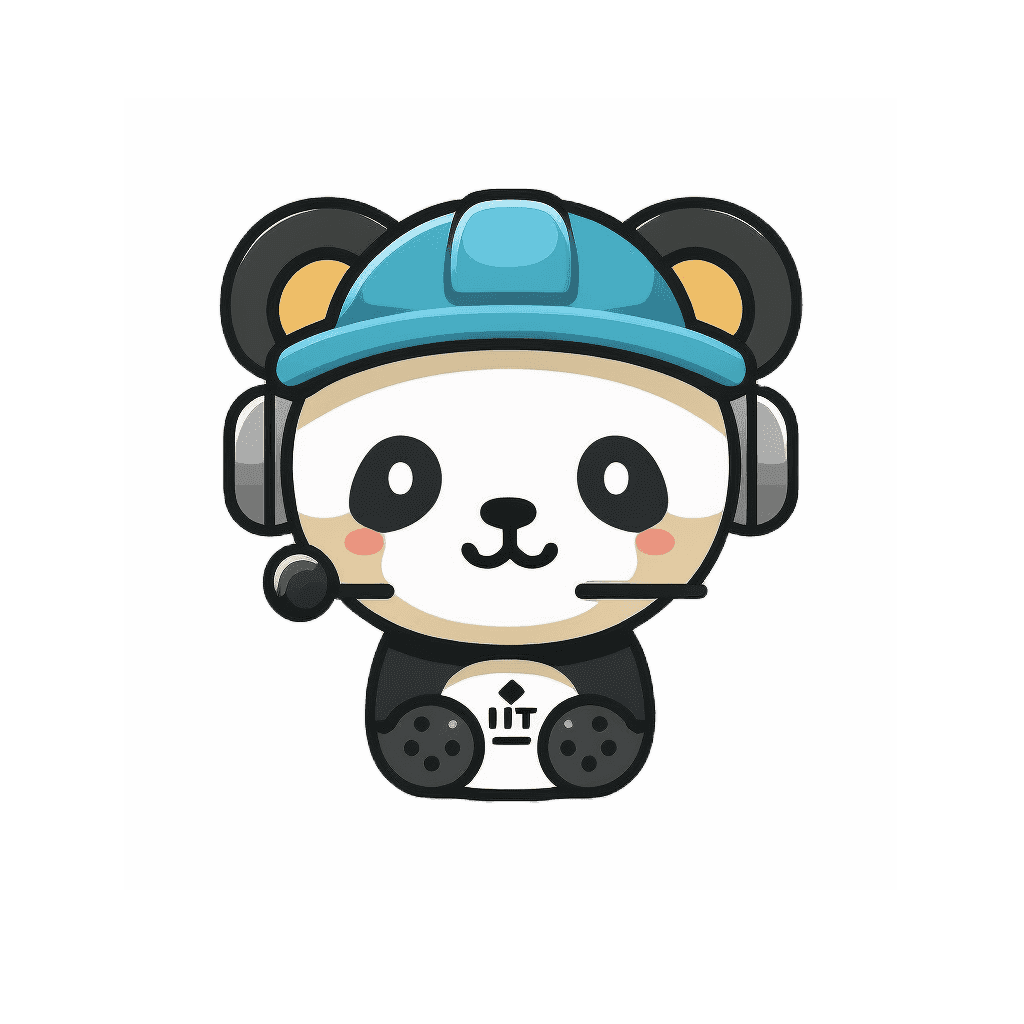